Predicting recoverable material stock in buildings: using machine learning with demolition audit data as a case study
Jul 17, 2023·,,
,·
0 min read
Natalia Kobylinska
Deepika Raghu
Matthew Gordon

Jens Hunhevicz
Catherine De Wolf
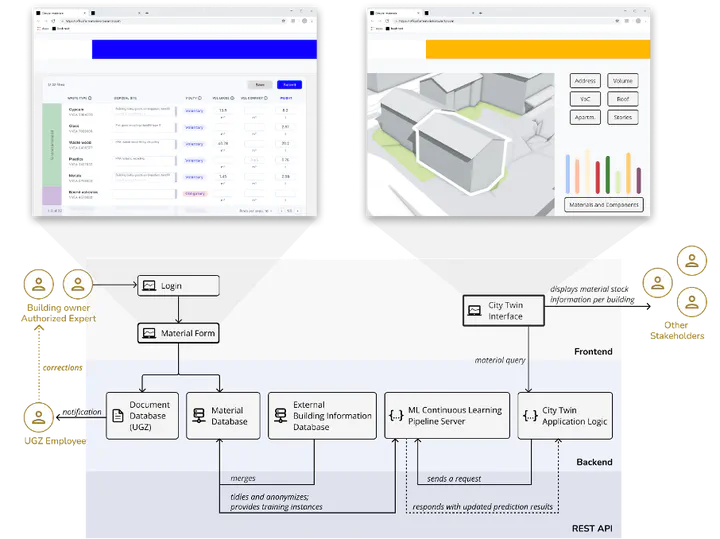
Abstract
Early specification of materials in buildings before their demolition could foster reuse in the construction industry. Studies have already shown the usefulness of machine learning in demolition waste estimation; however, application to real-world datasets is still limited. This study tests the feasibility of predicting recoverable material stock in the local context of the city of Zurich. The results show promise for the overall approach, although training models by using a small and heterogeneous dataset poses challenges. Therefore, we conceptualized an improved demolition data collection, processing, and dissemination. The resulting framework could help researchers and authorities in urban material stock estimation.
Type
Publication
Proceedings of the 2023 European Conference on Computing in Construction